(Part #10) Product matching via ML: The results
Previous topic: (Part #9) ML does work, but it’s not magic
Overall results did vary from one industry to another, from one client to another – is also very much depended on factors N (number of matching candidates) and M (the ease of match approval vs establishing a match fully manually) – but we were very happy to see the final result:
- The amount of work saved was ranging between 50% and 80%
- The overall project time was reduced between 45% and 75%
- We have delivered 100% accurate matches
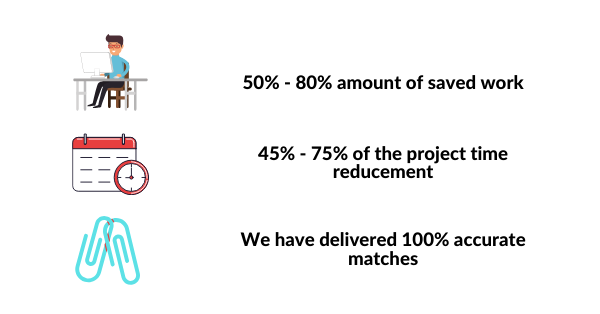
However, the gain was not universal, there were some important conditions that must be met in order for the ML process to make economic sense:
- Number of client’s product (Set A) would have to be over 10K (otherwise the ML data preparation overhead will be too big)
- The lower the expected matching rate is expected, the bigger the benefit of the ML process
- ML matching process is yet to be confirmed for non-western scripts (for example Chinese, Arabic, Russian)
- Products with variations (many products with different sizes, colours, or other types of variations) can be matched via ML, but they will negatively impact the ML benefit.
Lessons learned
Price2spy did not achieve the original goal we have set (full automation of the product matching process) – but we have found a viable alternative, and we have learned important lessons:
- Human work in product matching will be very difficult to eliminate. Instead, we should focus on decreasing it
- If used carefully, ML matching process can save between 50% and 80% amount of work and between 45% and 75% of the time needed for the project
- Data collected from competitors’ websites can be very unreliable (it has been populated by humans, after all), and needs QA and cleansing before feeding it into ML process
- Where applicable, Automatch is still superior to ML – simply because ML does require humans to do the final touch, while Automatch does not. The problem is – there are many cases where Automatch is not applicable
- ML product matching is superior to Hybrid Automatch
Of course, we’re not done with ML, nor with ML product matching. Right now, we’re working on the following:
- Checking ML process applicability on languages using non-Western scripts
- Further improving ML efficiency by adding the ability to compare product images (so far, our ML process was purely about text and numbers)
- Other lines of business where ML can be applied
We’ll keep you posted 🙂
Special thanks
My special thanks go to:
- Dušan Popović – External ML expert. Without Dušan’s expertise, we simply wouldn’t know where to start. He has set up foundation of our ML workflow and taught us valuable techniques and tricks (for example blocking). Dušan continued to contribute to the project even after migrating the project from Python to Java
- Andrija Kovačević – Price2Spy’s CTO. Andrija jumped on our ML project without a day of formal training, but after 18 months on the project, I count him as a serious ML authority.
- Sandra Tasić – head of Price2Spy’s manual product matching team. Sandra was quick to evaluate our ML experiments (even when the results were far from good), also at times when her hands were full of work to be done for clients.
- Nenad Đorđević and Jana Ćurčić – taken over the operational work from Andrija.
If you are interested to learn more, please visit these links:
Previous topic: (Part #9) ML does work, but it’s not magic