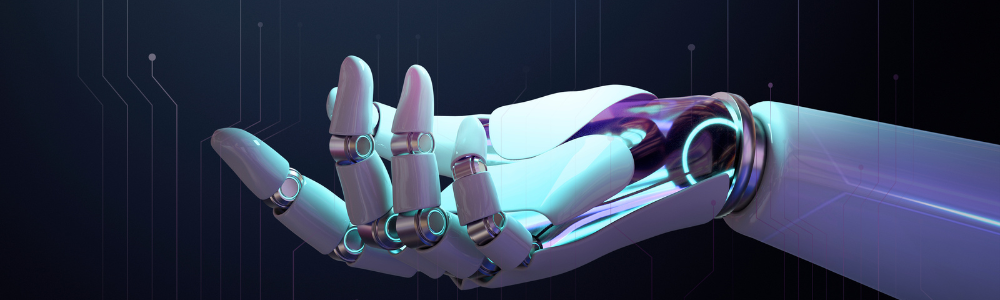
Machine learning in retail: How can retailers use it
Machine learning (ML) and Artificial intelligence (AI) are no more a novelty in the retail world. Machine learning in retail has been present for quite some time, especially in the case of big companies that rely on online sales.
However, it’s a common misconception that you need to be a big player who’s completely focused on online sales to benefit from machine learning.
Thanks to the versatility of machine learning in retail, retailers and brick-and-mortar stores of any size can improve their business.
Let’s see how.
What is machine learning in retail?
Customer preferences are an ever-shifting thing. Trends and behaviors are constantly changing, so to process huge data sets, identify patterns and get a deeper understanding, retailers need to rely on machine learning technologies.
The Fortune Business Insights predicted an increase of $5.84 billion in 2021 to $18.33 billion in 2028 year, making machine learning in retail the largest AI subset.
We mentioned that the usage of ML in the retail industry can be very versatile, but, indeed, it is mostly focused on improving online sales. Machine learning helps retailers to increase online sales in two main ways: by defining the best pricing strategy and by doing product matching.
- Pricing strategy
Each company has its pricing strategy objectives, but its pricing decisions can be seriously improved by using AI and ML technologies. Two main solutions that machine learning can offer are automatic pricing and pricing optimization.
Automated pricing means that ML helps define the product price by looking at data. The model focuses on finding the optimal price for each product, based on different factors such as product condition, category, description, historical pricing decision, and sales data, etc.
On the other hand, pricing optimization aims to understand how different customers react to different prices. Pricing optimization helps retailers estimate the right price for a certain product in a specific period. This further means that pricing optimization enables retailers to use dynamic pricing. This pricing model needs to forecast demand based on different factors such as seasonality, supply, and willingness to pay, just to name a few.
In the past, companies tried to do all this work by themselves. Moreover, they’ve done it manually. But, over time, everyone understood that’s mission impossible. That resulted in the appearance of numerous price monitoring tools that use machine learning technology to automate these processes.
- Product matching
Defining the best pricing strategy is not a one-sided thing. Besides knowing their product and customers, retailers must also know their competitors. One of the important factors in staying competitive is offering the best possible market price. To do that, retailers need to keep an eye on their competitors’ prices. That’s when product matching comes in.
Product matching is the process of recognizing identical or similar items in two or more competitors’ catalogs. When products get identified, their prices can also be compared and adjusted if needed. If we tell you that around 96% of customers compare prices before making a purchase decision, it’s clear that product matching and machine learning in retail are essential for better profitability.
Same as in the case of pricing optimization, product matching can, up to some point, be done manually. But, when the catalogs get too big, things start to get complicated. Therefore, this process is nowadays also automated.
ML and AI in Marketing
Machine learning in retail can be used in many ways, and one of them is in marketing. When one thinks about ML and AI, probably the first association is not marketing, but in reality, there are many aspects in which these two can be combined.
- Predicting customer behavior
This is one of the most important steps when it comes to organizing pricing and marketing strategies. With the help of machine learning, retailers can group customers into different segments, and make more personalized decisions. Those segments are based on the customer’s previous purchases and behavior patterns, meaning that retailers can detect a behavior pattern for each customer group.
Once the retailer understands the previous behavior, predicting the future becomes much easier. It’s needless to say that without understanding customer behavior, retailers can’t set the right prices and define suitable marketing strategies.
- Marketing campaign optimization
Like pricing strategies, marketing campaigns also require a deep market understanding. In both B2B and B2C industries, repeated buys are something that retailers are looking for. Machine learning in retail helps businesses to do better targeting when proposing recommendations. This is done by analyzing a huge amount of data and trying to define certain patterns. Moreover, machine learning helps with promotion optimization. To some extent, it’s similar to pricing optimization because it’s necessary to understand which prices would be the most profitable and then promote them accordingly.
Can brick-and-mortar retailers also use ML?
Yes, Contrary to popular belief, machine learning in retail is not only useful in online sales but in physical stores as well. The biggest advantage of physical stores is the interaction between humans and products that can’t be achieved in the online world. Those interactions provide valuable insights such as:
- Customer flow counting
Knowing the number of people that visit the store helps retailers to understand not only the conversion ratio but also the distribution of people that come in daily. The overall number of customers, as well as the peak hours, are not something to be neglected. By knowing this, retailers can decide on the number of staff they need, or when to offer special prices and promotions. Nowadays, machine learning in retail is often focused on computer vision algorithms that can help retailers with data collection and analysis.
- Understanding navigational routes
Another factor influencing purchasing behavior in physical stores is the position of items. Where to put different items? Are people focusing more on the products displayed on the front part of the store? Are they following a certain pattern when checking out the products? Are the customers’ age and sex important factors? Moreover, retailers can further enhance their operations by implementing retail scheduling management software. This software utilizes machine learning to optimize staff scheduling and ensure that the right number of employees are present during peak hours and special events, creating a more efficient and customer-focused in-store experience.
Wrapping it up
The retail industry keeps evolving and new ways of solving the challenges keep emerging almost daily. So far, machine learning in retail has made one of the biggest impacts on the industry. It helps improve many business aspects, so is no surprise that retailers are using it more frequently.
Are you thinking of integrating ML into your business? If that’s the case, we hope that our short guide will help you understand the ML benefits a bit better.
For those of you who are already familiar with this topic, and are interested in learning more about the technical aspects of machine learning and artificial intelligence, we invite you to check out the series of posts that we’ve done on the topic.
If you’re looking for ML solutions that can help you with pricing optimization, we invite you to check out Price2Spy and see for yourself why more over than 700 clients trust us.